Should SEOs Care About Internal Links? – Whiteboard Friday
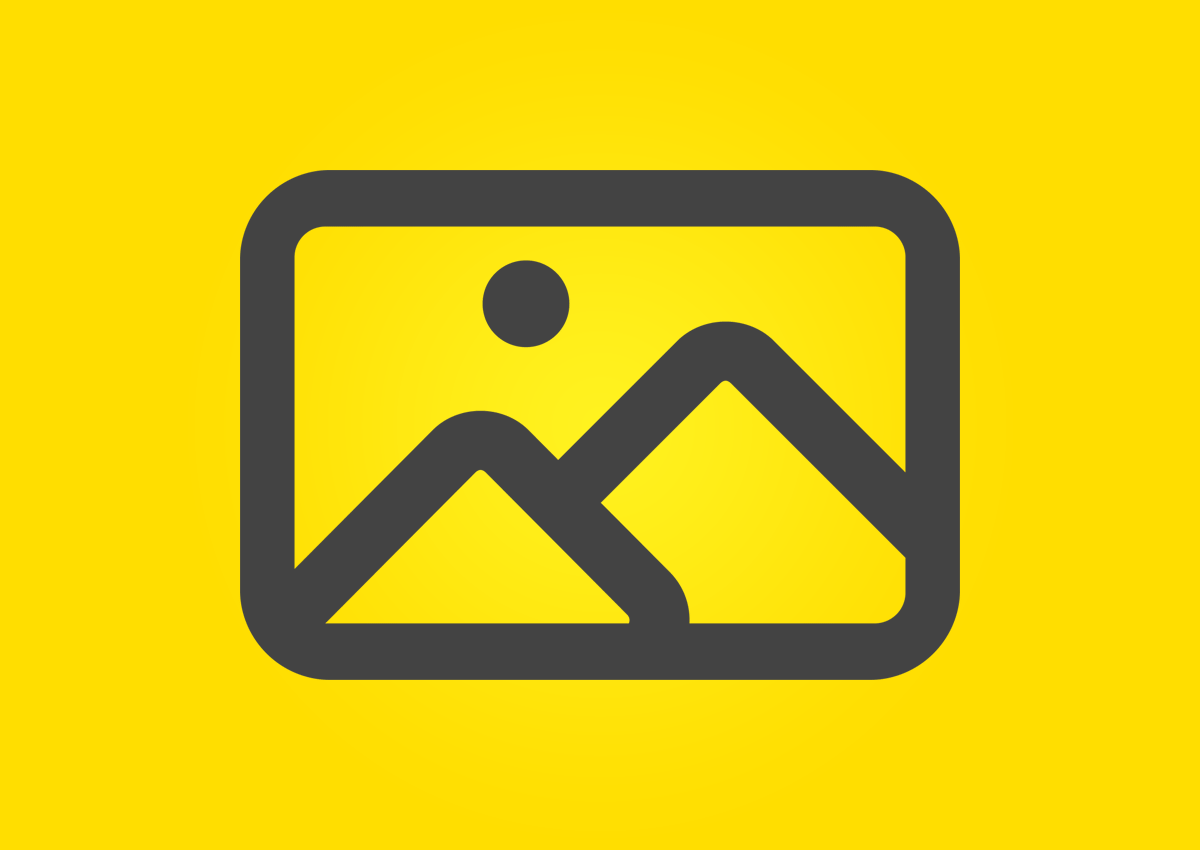
Posted by randfish
Internal links are one of those essential SEO items you have to get right to avoid getting them really wrong. Rand shares 18 tips to help inform your strategy, going into detail about their attributes, internal vs. external links, ideal link structures, and much, much more in this edition of Whiteboard Friday.
Click on the whiteboard image above to open a high-resolution version in a new tab!
Video Transcription
Howdy, Moz fans, and welcome to another edition of Whiteboard Friday. This week we’re going to chat a little bit about internal links and internal link structures. Now, it is not the most exciting thing in the SEO world, but it’s something that you have to get right and getting it wrong can actually cause lots of problems.
Attributes of internal links
So let’s start by talking about some of the things that are true about internal links. Internal links, when I say that phrase, what I mean is a link that exists on a website, let’s say ABC.com here, that is linking to a page on the same website, so over here, linking to another page on ABC.com. We’ll do /A and /B. This is actually my shipping routes page. So you can see I’m linking from A to B with the anchor text “shipping routes.”
The idea of an internal link is really initially to drive visitors from one place to another, to show them where they need to go to navigate from one spot on your site to another spot. They’re different from internal links only in that, in the HTML code, you’re pointing to the same fundamental root domain. In the initial early versions of the internet, that didn’t matter all that much, but for SEO, it matters quite a bit because external links are treated very differently from internal links. That is not to say, however, that internal links have no power or no ability to change rankings, to change crawling patterns and to change how a search engine views your site. That’s what we need to chat about.1. Anchor text is something that can be considered. The search engines have generally minimized its importance, but it’s certainly something that’s in there for internal links.
2. The location on the page actually matters quite a bit, just as it does with external links. Internal links, it’s almost more so in that navigation and footers specifically have attributes around internal links that can be problematic.
Those are essentially when Google in particular sees manipulation in the internal link structure, specifically things like you’ve stuffed anchor text into all of the internal links trying to get this shipping routes page ranking by putting a little link down here in the footer of every single page and then pointing over here trying to game and manipulate us, they hate that. In fact, there is an algorithmic penalty for that kind of stuff, and we can see it very directly.
We’ve actually run tests where we’ve observed that jamming this type of anchor text-rich links into footers or into navigation and then removing it gets a site indexed, well let’s not say indexed, let’s say ranking well and then ranking poorly when you do it. Google reverses that penalty pretty quickly too, which is nice. So if you are not ranking well and you’re like, “Oh no, Rand, I’ve been doing a lot of that,” maybe take it away. Your rankings might come right back. That’s great.
3. The link target matters obviously from one place to another.
4. The importance of the linking page, this is actually a big one with internal links. So it is generally the case that if a page on your website has lots of external links pointing to it, it gains authority and it has more ability to sort of generate a little bit, not nearly as much as external links, but a little bit of ranking power and influence by linking to other pages. So if you have very well-linked two pages on your site, you should make sure to link out from those to pages on your site that a) need it and b) are actually useful for your users. That’s another signal we’ll talk about.5. The relevance of the link, so pointing to my shipping routes page from a page about other types of shipping information, totally great. Pointing to it from my dog food page, well, it doesn’t make great sense. Unless I’m talking about shipping routes of dog food specifically, it seems like it’s lacking some of that context, and search engines can pick up on that as well.
6. The first link on the page. So this matters mostly in terms of the anchor text, just as it does for external links. Basically, if you are linking in a bunch of different places to this page from this one, Google will usually, at least in all of our experiments so far, count the first anchor text only. So if I have six different links to this and the first link says “Click here,” “Click here” is the anchor text that Google is going to apply, not “Click here” and “shipping routes” and “shipping.” Those subsequent links won’t matter as much.
7. Then the type of link matters too. Obviously, I would recommend that you keep it in the HTML link format rather than trying to do something fancy with JavaScript. Even though Google can technically follow those, it looks to us like they’re not treated with quite the same authority and ranking influence. Text is slightly, slightly better than images in our testing, although that testing is a few years old at this point. So maybe image links are treated exactly the same. Either way, do make sure you have that. If you’re doing image links, by the way, remember that the alt attribute of that image is what becomes the anchor text of that link.Internal versus external links
A. External links usually give more authority and ranking ability.
That shouldn’t be surprising. An external link is like a vote from an independent, hopefully independent, hopefully editorially given website to your website saying, “This is a good place for you to go for this type of information.” On your own site, it’s like a vote for yourself, so engines don’t treat it the same.
B. Anchor text of internal links generally have less influence.C. A lack of internal links can seriously hamper a page’s ability to get crawled + ranked.
It is, however, the case that a lack of internal links, like an orphan page that doesn’t have many internal or any internal links from the rest of its website, that can really hamper a page’s ability to rank. Sometimes it will happen. External links will point to a page. You’ll see that page in your analytics or in a report about your links from Moz or Ahrefs or Majestic, and then you go, “Oh my gosh, I’m not linking to that page at all from anywhere else on my site.” That’s a bad idea. Don’t do that. That is definitely problematic.
D. It’s still the case, by the way, that, broadly speaking, pages with more links on them will send less link value per link.
So, essentially, you remember the original PageRank formula from Google. It said basically like, “Oh, well, if there are five links, send one-fifth of the PageRank power to each of those, and if there are four links, send one-fourth.” Obviously, one-fourth is bigger than one-fifth. So taking away that fifth link could mean that each of the four pages that you’ve linked to get a little bit more ranking authority and influence in the original PageRank algorithm.
Look, PageRank is old, very, very old at this point, but at least the theories behind it are not completely gone. So it is the case that if you have a page with tons and tons of links on it, that tends to send out less authority and influence than a page with few links on it, which is why it can definitely pay to do some spring cleaning on your website and clear out any rubbish pages or rubbish links, ones that visitors don’t want, that search engines don’t want, that you don’t care about. Clearing that up can actually have a positive influence. We’ve seen that on a number of websites where they’ve cleaned up their information architecture, whittled down their links to just the stuff that matters the most and the pages that matter the most, and then seen increased rankings across the board from all sorts of signals, positive signals, user engagement signals, link signals, context signals that help the engine them rank better.
E. Internal link flow (aka PR sculpting) is rarely effective, and usually has only mild effects… BUT a little of the right internal linking can go a long way.
When PageRank was the dominant algorithm inside of Google’s ranking system, yeah, it was the case that PageRank sculpting could have some real effect. These days, that is dramatically reduced. It’s not entirely gone because of some of these other principles that we’ve talked about, just having lots of links on a page for no particularly good reason is generally bad and can have harmful effects and having few carefully chosen ones has good effects. But most of the time, internal linking, optimizing internal linking beyond a certain point is not very valuable, not a great value add.
But a little of what I’m calling the right internal linking, that’s what we’re going to talk about, can go a long way. For example, if you have those orphan pages or pages that are clearly the next step in a process or that users want and they cannot find them or engines can’t find them through the link structure, it’s bad. Fixing that can have a positive impact.Ideal internal link structures
I. No page should be more than 3 link “hops” away from another (on most small–>medium sites).
Now, the idea behind this is that basically in one, two, three hops, three links away from the homepage and three links away from any page on the site, I can get to up to a million pages. So when you talk about, “How many clicks do I have to get? How far away is this in terms of link distance from any other page on the site?” a great internal linking structure should be able to get you there in three or fewer link hops. If it’s a lot more, you might have an internal linking structure that’s really creating sort of these long pathways of forcing you to click before you can ever reach something, and that is not ideal, which is why it can make very good sense to build smart categories and subcategories to help people get in there.
I’ll give you the most basic example in the world, a traditional blog. In order to reach any post that was published two years ago, I’ve got to click Next, Next, Next, Next, Next, Next through all this pagination until I finally get there. Or if I’ve done a really good job with my categories and my subcategories, I can click on the category of that blog post and I can find it very quickly in a list of the last 50 blog posts in that particular category, great, or by author or by tag, however you’re doing your navigation.II. Pages should contain links that visitors will find relevant and useful.
If no one ever clicks on a link, that is a bad signal for your site, and it is a bad signal for Google as well. I don’t just mean no one ever. Very, very few people ever and many of them who do click it click the back button because it wasn’t what they wanted. That’s also a bad sign.
III. Just as no two pages should be targeting the same keyword or searcher intent, likewise no two links should be using the same anchor text to point to different pages. Canonicalize!
For example, if over here I had a shipping routes link that pointed to this page and then another shipping routes link, same anchor text pointing to a separate page, page C, why am I doing that? Why am I creating competition between my own two pages? Why am I having two things that serve the same function or at least to visitors would appear to serve the same function and search engines too? I should canonicalize those. Canonicalize those links, canonicalize those pages. If a page is serving the same intent and keywords, keep it together.
IV. Limit use of the rel=”nofollow” to UGC or specific untrusted external links. It won’t help your internal link flow efforts for SEO.
Rel=”nofollow” was sort of the classic way that people had been doing PageRank sculpting that we talked about earlier here. I would strongly recommend against using it for that purpose. Google said that they’ve put in some preventative measures so that rel=”nofollow” links sort of do this leaking PageRank thing, as they call it. I wouldn’t stress too much about that, but I certainly wouldn’t use rel=”nofollow.”
What I would do is if I’m trying to do internal link sculpting, I would just do careful curation of the links and pages that I’ve got. That is the best way to help your internal link flow. That’s things like…V. Removing low-value content, low-engagement content and creating internal links that people actually do want. That is going to give you the best results.
VI. Don’t orphan! Make sure pages that matter have links to (and from) them. Last, but not least, there should never be an orphan. There should never be a page with no links to it, and certainly there should never be a page that is well linked to that isn’t linking back out to portions of your site that are of interest or value to visitors and to Google.
So following these practices, I think you can do some awesome internal link analysis, internal link optimization and help your SEO efforts and the value visitors get from your site. We’ll see you again next week for another edition of Whiteboard Friday. Take care.
Video transcription by Speechpad.com
Sign up for The Moz Top 10, a semimonthly mailer updating you on the top ten hottest pieces of SEO news, tips, and rad links uncovered by the Moz team. Think of it as your exclusive digest of stuff you don’t have time to hunt down but want to read!
Continue reading →